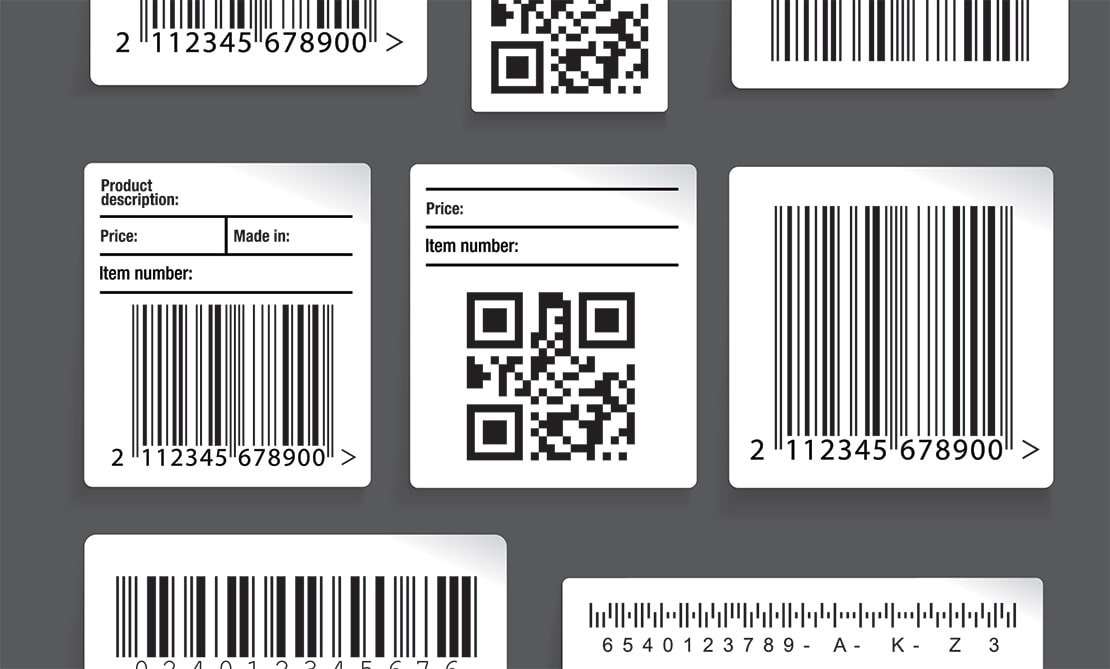
Why Bad Product Data Disrupts eCommerce Implementations
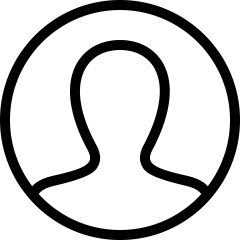
There are many challenges in “getting it right” when it comes to product data, and we will dive into this topic in the context of an eCommerce implementation. This may be a refresher for some, or a fair warning to others that may not have the experience, but in either case it should provide valuable information to help eCommerce implementation teams prepare for a project and reduce downstream headaches, by prioritizing and planning for these issues earlier in the project.
Sources of Product Data and the Importance of Enabling a Commerce Experience
Sources are vast when it comes to product data. The most common source is a company’s ERP system where the master data must live so customers can properly place orders. This data is usually minimal and consists of the product ID, product name and maybe a short description (usually not customer facing). This is the foundation for product data, but certainly won’t accurately represent all of the relevant information to the customer.
That is where the many other sources come into play. These include (but are not limited to): manufacturer data, third party data (such as product reviews, tech specs), marketing authored product content, digital assets, and other generic unstructured data. This disparate data can lead to the following:
Formatting differences of the same product attributes
Variations to the product ID
Duplicate information
Missing data
Broken references such as hyperlinks or imagery
Enabling an eCommerce experience requires sound product data management and governance. Usually a key component during an implementation is normalizing and merging the product data from these various sources. For example, if the ERP has a short description that is brief and formatted in all caps, but a marketing authored short description available, then the marketing authored short description should be utilized for the website. This merged product data will act as the “digital product master” in order to serve up the same enriched product content to all touch-points for the customer.
Impact on Customer Experience
Normalized and consolidated product data is critical for a consistent end-consumer experience and is paramount in enabling them to make informed purchasing decisions. This data is utilized on product listing pages to help the consumer find relevant products. Dynamic search and navigation capabilities are only as good as the underlying data!
Once the consumer drills into a specific product detail page, this data will help inform the consumer and give them confidence that they are purchasing the correct product. Whether it’s technical specifications, user manuals or fit guides, this is essential to the customer proceeding with a transaction versus abandoning their purchase.
Finally, the product data is utilized through the remainder of the buy-flow to present relevant data to the customer while making a purchase.
Isn’t all of this obvious?
Yes, but time and time again, product data is in a compromising position where these seemingly straightforward website capabilities are completely degraded because the right measures have not been put in place to make the completeness and accuracy of product data a priority.
Where Product Data Falls Flat
Now that we’ve aligned on the variety of product data sources and their importance in enabling an eCommerce experience, we will detail the common pitfalls and “gotchas” surrounding that product data. These are the red flags to be aware of during an eCommerce buildout, regardless of who is responsible for the work.
Data Cleanliness
First and foremost, the cleanliness of existing product data often poses big problems. Companies tend to leave data untouched for years, and fail to keep it current. Not necessarily the dynamic data, like pricing or inventory, but rather the authored attributes of products. There could be: key attributes missing, product descriptions that are internal facing and not customer facing, or products that should have been deactivated years ago, etc. During the project, companies realize that there are a lot of problems that have accumulated over time – and usually they are not staffed to retroactively clean up the data.
In addition to cleanliness of data, lack of product data required to support the customer experience is a big challenge. Given the needs for product detail and product listing pages noted above, existing data usually needs to be supplemented with additional attribution and content, or restructured in order to enable what the business wants. This could require initiatives to get the weights and dimensions of products, hiring a copywriter for web descriptions, adding colors & sizes, creating translations for all of the marketing product content, etc.
Basically, the current team makeup across multiple business units is unlikely to be staffed to support this initiative, nor is it likely that there is governance and processes to properly enable this set of activities. It’s then a question of prioritization and how much of this gap will be closed in the allotted time.
Data Availability
For some reason, companies tend to have difficulty extracting and aggregating all of their existing product data. When building a new site, the design of the solution is based around real data. When the reliance shifts to the QA team and development team to mock up sample data to support local testing, it increases risk for future quality issues. There is always a need to obtain a sample product data file from the client very early in the project, prior to development. It is a rarity that it is delivered that early, if at all.
You can see how this can start to compound. Data is stale, data is not complete, and delivery of real data to the implementation team is delayed. So when the data does finally get loaded, issues are uncovered. As a result, the experience can be really compromised, leading to a late realization that there is a ton of work required to make it work. And from there, fixing the data is de-prioritized in order to get the site live, and the cycle continues. Bad, stale, inaccurate data is persisted to the next big implementation…
Knowledge and Ownership
Surrounding all of these data related pitfalls, is the lack of historical knowledge related to the product data. Employees tend to turn over, and the core knowledge for the products goes with them as well. Understanding master data system of record, product code naming conventions, taxonomy structure, and what is relevant or not within the existing product data is critical to optimizing the web experience. There can be a huge learning curve for employees on this front, which combined with an aggressive project schedule can be a significant challenge.
Even though these product data culprits can hinder your implementation, they can be overcome with the right planning and coordination.
Lessons Learned and Keys to Success
These pitfalls have all surfaced on many projects over the years, and as a result, there are ways to mitigate these issues and enable a successful project without letting issues with product data derail the project. Here are the keys to success we’ve tried to instill on projects, after seeing these issues consistently arise:
1. Focus on the customer! Bad product data cannot be a justification for a degraded user experience
Customer experience should be the driver for data, not the other way around. The sooner this is understood, realized, and incorporated on the business team’s track of work is essential. In order to enable what’s necessary, often times that means that “net new” content needs to be provided. The crutch on projects is to say “we are not staffed to support this” or “it’s always been this way and we are treating this as ‘like-for-like'”. It’s sometimes a hard sell, so the focus should really be on the business case behind it. The business case can vary, but could revolve around reduced call volume to customer service, reduced return rate, brand loyalty, increased conversions, etc.
Secondly, what do the customers really need? Understanding and focusing on the needs of the customers will help with the closure of any product data gaps, so that the hard work and effort is very tangible and can easily be validated by users. Have a focus on the prioritization that helps meet the needs of the majority of the consumers.
Additionally, executive sponsors are likely the last to know about this poor product data situation. Do you really think that they are going to be happy when they find out that the team skipped over the work required and now there is a shoddy user experience as a result? No. And that’s not a conversation that is desirable after a multi-million dollar project just took place.
2. Good product data doesn’t happen overnight – deal with it and plan for it
Product data work streams should be started on Day 1 of any eCommerce project. The lead time is necessary to get the right resources in place and plan out the tasks required. Those tasks can include:
Gap analysis of current data v. data required to support the new experience
Export of existing data
Data migration (including cleanup – no reason to migrate bad data)
Assignment of owners – accountability is extremely important
Etc.
Planning these items is no easy task. The reality is that the implementation team likely does not have ownership of these tasks in their scope of work. Helping with the focus and planning, and identifying a lot of these “gotchas” for the client is best for all parties involved. This is key in leading to a more successful implementation, rather than the common “not my problem” approach.
3. Find the tribal knowledge
In order to address some of the data problems we’ve talked about, understanding the products and knowing what the data means, is very important. This is often a big challenge, and difficult to overcome. Usually though, someone in the organization is the subject matter expert and can provide the answers. Sometimes that person is on the IT side responsible for product data entry in the ERP, or maybe that person is focused on the supply chain side where the product data like weights and dimensions may be the key elements. It’s just a matter of finding that individual and getting them engaged on the project – which is why it is critical to have executive-level support of these types of initiatives, as the necessary resources likely span multiple business units that may be outside the individual project’s control.
In Summary
We’ve detailed the importance of product data, what we usually see go awry, and the keys to success in making sure that the product data enablement is successful. Planning for product data migration from the start will dramatically increase your chances for a successful implementation and lead to a positive response from your end-consumers.