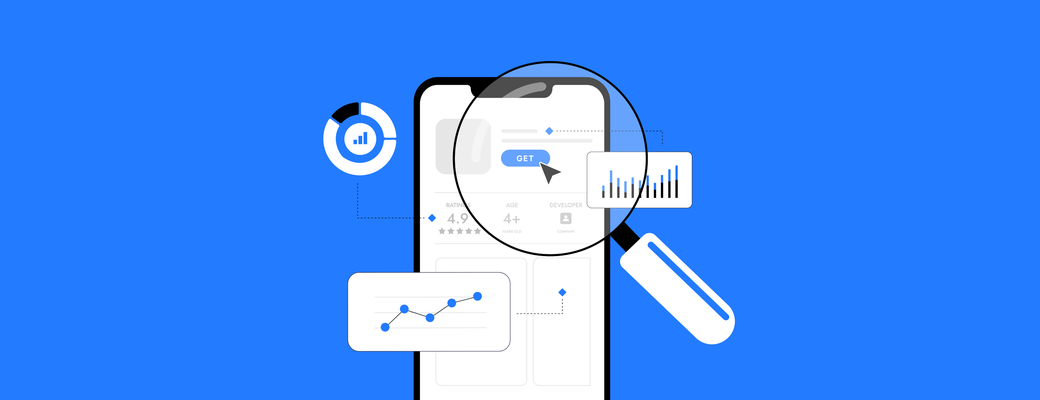
Understanding SAN vs. Non-SAN Attribution & Why the Difference Matters
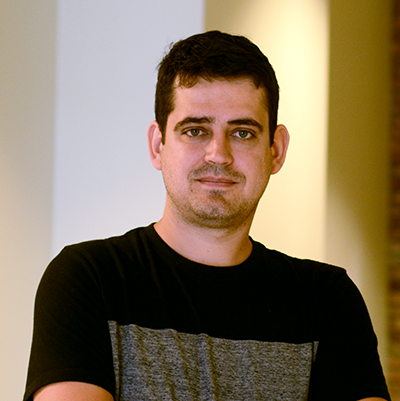
If you’ve spent any time running acquisition campaigns for a mobile app, you've probably noticed that attribution data can look slightly different across ad platforms. That’s to be expected—it’s a byproduct of how those platforms communicate with your app or mobile measurement platform. The core reason lies in whether the ad platform operates as a Self-Attributing Network (SAN) or a Non-Self-Attributing Network (Non-SAN).
Understanding the distinction is critical to interpreting your campaign performance accurately and making smart marketing decisions. In this post, we’ll help you better understand the differences between the two types of platforms, and offer some guidance of how you can apply this information to your own analysis.
What is a Self-Attributing Network?
A Self-Attributing Network (SAN) is an ad platform that handles attribution internally. When a user converts on your app, your system sends a signal to the SAN asking, “Was this your user?” If the SAN recognizes the user, it will send attribution data back “claiming” them.
Rather than relying on tracking links for attribution, SAN platforms receive user metadata from your system, then compare it to the information they’ve stored about user impressions and clicks to determine if the user came from their platform. This metadata will typically include a number of data points, such as the mobile identifier ID (IDFA/GAID), device details, conversion timestamp, and more.
Some of the biggest players in mobile advertising are SANs. These include:
Meta Ads
Google Ads
TikTok
Apple Search Ads
Snapchat
Since these platforms function as black boxes—providing limited visibility into click and impression data—you may encounter situations where multiple platforms ‘claim' the same user conversion, leading to attribution discrepancies in your reports.

What is a Non-SAN Platform?
A Non-SAN platform doesn’t self-attribute. Instead, they rely on tracking links to tie user behavior back to a specific campaign. When setting up your campaigns, you’ll be asked to provide a unique impression or click tracking link that embeds key information about the user. Then, when an impression or click occurs, the ad platform will send a ping to your system with the information provided in the tracking link.
From there, your system records the click/impression along with its associated user data, and later compares it against conversion details to handle attribution.
Many ad networks fall into the Non-SAN category, including:
Pinterest
Reddit
Outbrain
Because your systems plays a more central role in data collection, Non-SAN platforms provide greater data transparency and reduce attribution overlap across channels.

Difference between SAN and Non-San Platforms
It may be helpful to think of the differences between SAN and Non-SAN platforms in terms of features. The table below covers the most crucial aspects of mobile measurement, and how they stack up between the two types of platforms.
| SAN | Non-SAN |
Attribution Control | Ad platform self-attributes conversions and informs your data systems | App-side data platform fully controls attribution using tracking links |
Click Tracking | Clicks are not shared with the advertiser | Click data is recorded via tracking links |
Impression Tracking | Impressions are not shared with the advertiser | Impressions data is recorded via tracking links |
Postback Flow | App requests attribution from the SAN; SAN decides whether to claim the conversion | App or data service sends postbacks to the ad platform with full attribution details |
Tracking Links | Not allowed (SANs track clicks internally) | Fully Supported (tracking links are used in ads) |
Deep Linking | Supported but requires additional setup using SAN deep linking solution | Fully supported using tracking links |
Data Transparency | Limited (SANs do not share raw click/impression data) | High transparency (app-side data platform has access to all tracked data) |
SKAN Attribution | Always applies for iOS traffic | Default to SKAN if consent is not granted, but app can also receive IDFA if user consents |
IDFA/GAID Access | IDFA/GAID is generally withheld (even if user consents to ATT). | App can receive IDFA if user consents to ATT. GAID is available. |
How is Attribution Impacted?
The distinction between SAN and Non-SAN platforms has a significant impact on how attribution is handled. Here’s a quick rundown of the strengths and weaknesses of each:
Strengths of SAN Attribution:
Simplicity & Integration: SANs reduce setup complexity and minimizing reliance on tracking links, which can break or be blocked by privacy tools.
Real-Time Optimizations: Since SANs have direct access to their own ecosystem data, they can offer real-time campaign adjustments that improve ad performance.
Privacy Compliance: SANs are designed to align with evolving privacy standards, reducing the burden on advertisers to manage compliance.
Challenges with SANs:
Attribution Overlap: SANs may claim conversions even if another channel influenced the user, leading to double-attribution or credit-stealing across platforms.
Data Transparency: Click and impression data remain within the SAN’s ecosystem, limiting visibility for cross-channel analysis, custom attribution models, data validation, and discrepancy resolution.
Strengths of Non-SANs:
Greater Data Control: Non-SAN platforms rely on tracking links, giving advertisers and MMPs more visibility into user journeys, clicks, and impressions.
Custom Attribution Models: With fuller datasets, businesses can implement multi-touch or custom attribution frameworks tailored to their needs.
Challenges with Non-SANs:
Tracking Link Risks: Tracking links require more hands-on management, are prone to human error. and can be blocked by browser restrictions, ad blockers, or impacted by privacy changes—particularly on iOS.
Latency & Optimization: Since Non-SANs depend on external attribution systems, there can be delays in data processing, which may affect real-time optimization capabilities.
Ultimately, neither approach is inherently better—it depends on your business goals, technical resources, and privacy considerations. Many advertisers adopt a hybrid strategy, leveraging the strengths of both SAN and Non-SAN platforms while using an MMP to unify reporting and mitigate attribution challenges.
Conclusion
It’s crucial to understand how your data is collected in any context, but that’s especially true when you’re dealing with attribution data and your mobile ad partners. While your options for improving any data collection shortcomings is limited, you do have some options for improving your mobile attribution modeling. Consider, for example, a Mobile Measurement Platform (MMP) such as AppsFlyer or Adjust. These tools typically offer machine learning models and multi-channel attribution features to offset the data you’re lacking and help you make better marketing decisions.
If you have any questions about the mobile ad platforms you’re using, or need help making sense of the data you have, we hope you’ll drop us a line.