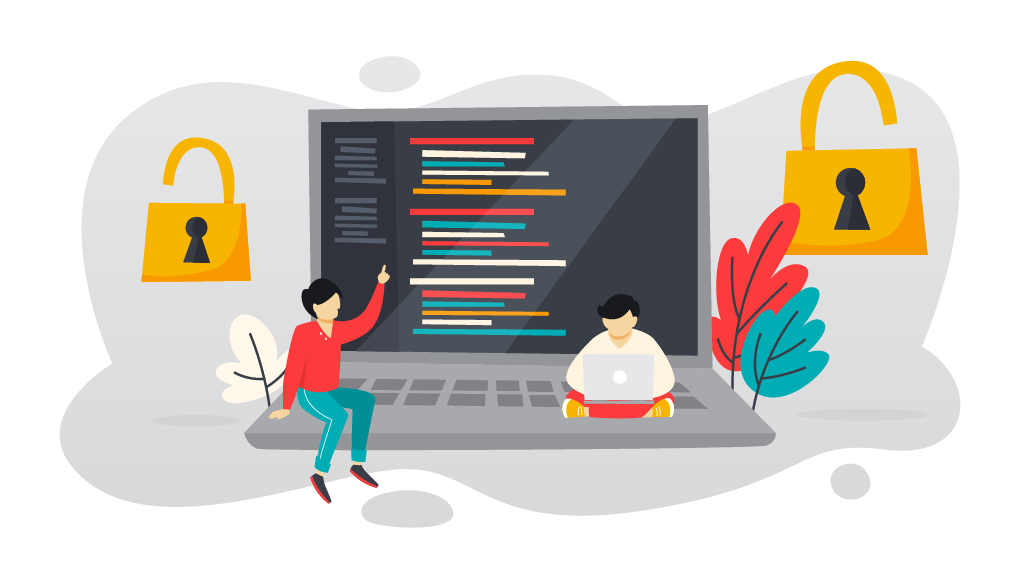
Giving Back: Lessons in Open Source
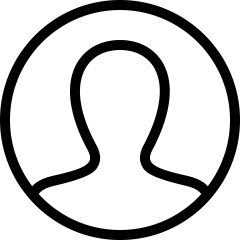
Our Open Source Story
As an organization, Avatria has always admired the advancements made to the software development community via open-source technology. Back in 2015 we kicked off our first open-source project, called “ydeploy,” an SAP Hybris build and deployment automation tool. But since then, between client work, product development, hiring, and other internal initiatives, we’ve found it difficult to contribute.
Last Fall, the Avatria team decided to change that. We wanted to get back to contributing to the open-source world for a few reasons:
We’ve relied on other people’s code countless times over the years, and we wanted to give back to the community.
It’s a great way to develop new technical skills, keep up our coding chops, and learn new skills.
We’d be lying if we pretended that it isn’t a good look for the company—it gets our name out there and shows off our engineering skills.
As we started to brainstorm where to focus our energies, we realized we had a perfect opportunity sitting right in front of us. Our Data Science team had been using LightGBM (an open-source library itself!) as one of the machine learning frameworks behind our Convert product, and while it was a great fit for many of our purposes, we’d also been running into some constraints. To make a long story short, the datasets we were using for training were taxing the memory available to our servers, and we didn’t want to compromise the quality of our results by downsampling or limiting the datasets we could work with.
This led us to Dask (another open-source library!), a computing framework that distributes data across clusters, reducing memory requirements. Although Dask already contained LightGBM support, unfortunately it was restricted to regression and classifier models, not the learning-to-rank models we rely upon.
The situation was perfect. The needs of our internal development (extending dask-lightgbm to support LTR models) dovetailed exactly with a gap in the existing open-source library.
The Review Process
A couple weeks after finishing our development and submitting a pull request (PR) to the dask-lightgbm GitHub repository, the project maintainer informed us that the package was being deprecated. However, the reasons for this were fortuitous: dask-lightgbm was being incorporated directly into the LightGBM library! We were put in touch with a LightGBM core maintainer, who asked that we migrate our changes directly to LightGBM. This was great news—our contribution would be merged into the much bigger and more actively supported LightGBM project, as part of its 3.2.0 release that included support for a new Dask module.
The code review process itself was a great learning opportunity. As a broadly used library, LightGBM has strong integration tests and a specific code style. Bringing our commits into alignment with both required additional development, in some cases beyond the scope of our original changes. This was exactly the outcome we’d hoped for when we re-started our open-source initiative: pushing our skillset further, and learning from developers outside of our walls.
Open Source Best Practices
If you’re looking to make your own contributions to the open-source community, here are some tips for making your first project a success.
Choose wisely: Unless an idea arises out of a project you’re actively working on, most pull requests actually originate as an issue on GitHub. If you’re new to open source, keep an eye out for issues tagged “Good first issue” or “Good for beginner.” If you’d like to take on the issue, leave a comment indicating that you would like to address it, and then it should be assigned to you.
Communicate first: Ask the maintainers whether they would like a PR for the issues you’re attempting to resolve before submitting the pull request. Submitting a PR out of the blue without reaching out to the maintainer(s) beforehand might not work out for the following reasons:
The maintainer(s) may have deliberately chosen not to support the functionality you’ve added in your pull request.
You may have submitted the pull request to a repository that is no longer being maintained.
The maintainer(s) may simply not get back to you. Open source maintainers tend to have busy lives and maintain projects for free.
Add unit tests: Nothing can assure an open source maintainer that your code will actually work more than thorough unit tests. It’s worth the extra time, and may save you from having to implement them later on.
Have patience: Your code could end up in the hands of dozens, hundreds, or thousands of users, and it’s worth making the small, syntactic changes that the maintainers ask for. Since a lot of open-source maintainers dedicate their time for free, consider sponsoring projects or individual contributors.
Conclusion With our first LightGBM pull request under our belt, we’ve got the open-source bug. We plan on continuing to contribute to LightGBM in the future, and have already submitted additional PRs for new enhancements we’ve been using internally. It’s exciting to put your code in the hands of other developers, and see where else it may go.
That said, Avatria’s commitment to open source does not end at our machine learning library of choice—we’ve started looking at our other projects to see if there are other opportunities to make public contributions. Keep your eye on this space; we hope you’ll see more open source commits, more of our own code, and more findings from our various research efforts.